What is one hot encoding in tensorflow?2019 Community Moderator ElectionTensorflow - How to set gradient of an external process (py_func)?Tensor Decomposition in TensorFlow for multinomial time series dimensionality reductionhow can I solve label shape problem in tensorflow when using one-hot encoding?RGB + Depth Encoding for CNNsReading a CSV in TensorFlow RNNOne hot encoding vs Word embeddingHow does one go about feature extraction for training labelled tweets for sentiment analysis?GAN with Conv2D using TensorFlow - Shape errorwhat is the one hot encoding for cancer data classificationHow to run a saved TensorFlow Model? (Video Prediction Model)
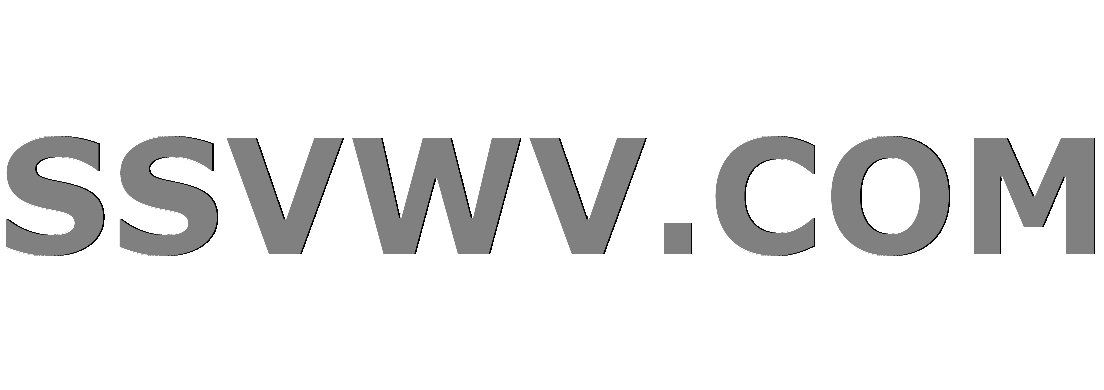
Multi tool use
Is it important to consider tone, melody, and musical form while writing a song?
Service Entrance Breakers Rain Shield
How is it possible to have an ability score that is less than 3?
Email Account under attack (really) - anything I can do?
What defenses are there against being summoned by the Gate spell?
Why did the Germans forbid the possession of pet pigeons in Rostov-on-Don in 1941?
To string or not to string
Why are 150k or 200k jobs considered good when there are 300k+ births a month?
How do I create uniquely male characters?
Why "Having chlorophyll without photosynthesis is actually very dangerous" and "like living with a bomb"?
How much RAM could one put in a typical 80386 setup?
How does strength of boric acid solution increase in presence of salicylic acid?
What is the offset in a seaplane's hull?
TGV timetables / schedules?
Can divisibility rules for digits be generalized to sum of digits
The Two and the One
Show that if two triangles built on parallel lines, with equal bases have the same perimeter only if they are congruent.
Why not use SQL instead of GraphQL?
Is it unprofessional to ask if a job posting on GlassDoor is real?
"You are your self first supporter", a more proper way to say it
How did the USSR manage to innovate in an environment characterized by government censorship and high bureaucracy?
Why don't electron-positron collisions release infinite energy?
What do the dots in this tr command do: tr .............A-Z A-ZA-Z <<< "JVPQBOV" (with 13 dots)
Why doesn't Newton's third law mean a person bounces back to where they started when they hit the ground?
What is one hot encoding in tensorflow?
2019 Community Moderator ElectionTensorflow - How to set gradient of an external process (py_func)?Tensor Decomposition in TensorFlow for multinomial time series dimensionality reductionhow can I solve label shape problem in tensorflow when using one-hot encoding?RGB + Depth Encoding for CNNsReading a CSV in TensorFlow RNNOne hot encoding vs Word embeddingHow does one go about feature extraction for training labelled tweets for sentiment analysis?GAN with Conv2D using TensorFlow - Shape errorwhat is the one hot encoding for cancer data classificationHow to run a saved TensorFlow Model? (Video Prediction Model)
$begingroup$
I am currently doing a course in tensorflow in which they used tf.one_hot(indices, depth). Now I don't understand how these indices change into that binary sequence.
Can somebody please explain to me the exact process???
machine-learning python neural-network deep-learning tensorflow
$endgroup$
add a comment |
$begingroup$
I am currently doing a course in tensorflow in which they used tf.one_hot(indices, depth). Now I don't understand how these indices change into that binary sequence.
Can somebody please explain to me the exact process???
machine-learning python neural-network deep-learning tensorflow
$endgroup$
add a comment |
$begingroup$
I am currently doing a course in tensorflow in which they used tf.one_hot(indices, depth). Now I don't understand how these indices change into that binary sequence.
Can somebody please explain to me the exact process???
machine-learning python neural-network deep-learning tensorflow
$endgroup$
I am currently doing a course in tensorflow in which they used tf.one_hot(indices, depth). Now I don't understand how these indices change into that binary sequence.
Can somebody please explain to me the exact process???
machine-learning python neural-network deep-learning tensorflow
machine-learning python neural-network deep-learning tensorflow
edited 35 mins ago
thanatoz
asked Apr 12 '18 at 9:42


thanatozthanatoz
569319
569319
add a comment |
add a comment |
2 Answers
2
active
oldest
votes
$begingroup$
Suppose you have a categorical feature in your dataset (e.g. color). And your samples can be either red, yellow or blue. In order to pass this argument to a ML algorithm, you first need to encode it so that instead of strings you have numbers.
The easiest way to do such a thing is to create a mapping where:
red --> 1
yellow --> 2
blue --> 3
and replace each string with its mapped value.
However this might create unwanted side effects in our ML model as when dealing with numbers it might think that blue > yellow (because 3 > 2) or that red + yellow = blue (because 1 + 2 = 3). The model has no way of knowing that these data were categorical and then were mapped as integers.
The solution to this problem is one-hot encoding where we create N new features, where N is the number of unique values in the original feature. In our exampel N would be equal to 3, because we have 3 unique colors (red, yellow and blue).
Each of these features be binary and would correspond to one of these unique values. In our example the first feature would be a binary feature telling us if that sample is red or not, the second would be the same thing for yellow and the third for blue.
An example of such a transformation is illustrated below:
Note, that because this approach increases the dimensionality of the dataset, if we have a feature that takes many unique values, we may want to use a more sparse encoding (like the one I presented above).
$endgroup$
add a comment |
$begingroup$
depth: A scalar defining the depth of the one hot dimension.
indices: A Tensor of indices.
This the example given in tensorflow documentation.
1. Only Specifying indices and depth(Default Values of on_value is 1 and off_value is 0)
indices = [0, 1, 2]
depth = 3
tf.one_hot(indices, depth) # output: [3 x 3]
# [[1., 0., 0.],
# [0., 1., 0.],
# [0., 0., 1.]]
- Specifying on_value and off_value
indices = [0, 2, -1, 1]
depth = 3
tf.one_hot(indices, depth, on_value=5.0, off_value=0.0, axis=-1)
##output: [4 x 3]
# [[5.0, 0.0, 0.0], # one_hot(0)
# [0.0, 0.0, 5.0], # one_hot(2)
# [0.0, 0.0, 0.0], # one_hot(-1)
# [0.0, 5.0, 0.0]] # one_hot(1)
You can also see the code on GitHub
$endgroup$
add a comment |
Your Answer
StackExchange.ifUsing("editor", function ()
return StackExchange.using("mathjaxEditing", function ()
StackExchange.MarkdownEditor.creationCallbacks.add(function (editor, postfix)
StackExchange.mathjaxEditing.prepareWmdForMathJax(editor, postfix, [["$", "$"], ["\\(","\\)"]]);
);
);
, "mathjax-editing");
StackExchange.ready(function()
var channelOptions =
tags: "".split(" "),
id: "557"
;
initTagRenderer("".split(" "), "".split(" "), channelOptions);
StackExchange.using("externalEditor", function()
// Have to fire editor after snippets, if snippets enabled
if (StackExchange.settings.snippets.snippetsEnabled)
StackExchange.using("snippets", function()
createEditor();
);
else
createEditor();
);
function createEditor()
StackExchange.prepareEditor(
heartbeatType: 'answer',
autoActivateHeartbeat: false,
convertImagesToLinks: false,
noModals: true,
showLowRepImageUploadWarning: true,
reputationToPostImages: null,
bindNavPrevention: true,
postfix: "",
imageUploader:
brandingHtml: "Powered by u003ca class="icon-imgur-white" href="https://imgur.com/"u003eu003c/au003e",
contentPolicyHtml: "User contributions licensed under u003ca href="https://creativecommons.org/licenses/by-sa/3.0/"u003ecc by-sa 3.0 with attribution requiredu003c/au003e u003ca href="https://stackoverflow.com/legal/content-policy"u003e(content policy)u003c/au003e",
allowUrls: true
,
onDemand: true,
discardSelector: ".discard-answer"
,immediatelyShowMarkdownHelp:true
);
);
Sign up or log in
StackExchange.ready(function ()
StackExchange.helpers.onClickDraftSave('#login-link');
);
Sign up using Google
Sign up using Facebook
Sign up using Email and Password
Post as a guest
Required, but never shown
StackExchange.ready(
function ()
StackExchange.openid.initPostLogin('.new-post-login', 'https%3a%2f%2fdatascience.stackexchange.com%2fquestions%2f30215%2fwhat-is-one-hot-encoding-in-tensorflow%23new-answer', 'question_page');
);
Post as a guest
Required, but never shown
2 Answers
2
active
oldest
votes
2 Answers
2
active
oldest
votes
active
oldest
votes
active
oldest
votes
$begingroup$
Suppose you have a categorical feature in your dataset (e.g. color). And your samples can be either red, yellow or blue. In order to pass this argument to a ML algorithm, you first need to encode it so that instead of strings you have numbers.
The easiest way to do such a thing is to create a mapping where:
red --> 1
yellow --> 2
blue --> 3
and replace each string with its mapped value.
However this might create unwanted side effects in our ML model as when dealing with numbers it might think that blue > yellow (because 3 > 2) or that red + yellow = blue (because 1 + 2 = 3). The model has no way of knowing that these data were categorical and then were mapped as integers.
The solution to this problem is one-hot encoding where we create N new features, where N is the number of unique values in the original feature. In our exampel N would be equal to 3, because we have 3 unique colors (red, yellow and blue).
Each of these features be binary and would correspond to one of these unique values. In our example the first feature would be a binary feature telling us if that sample is red or not, the second would be the same thing for yellow and the third for blue.
An example of such a transformation is illustrated below:
Note, that because this approach increases the dimensionality of the dataset, if we have a feature that takes many unique values, we may want to use a more sparse encoding (like the one I presented above).
$endgroup$
add a comment |
$begingroup$
Suppose you have a categorical feature in your dataset (e.g. color). And your samples can be either red, yellow or blue. In order to pass this argument to a ML algorithm, you first need to encode it so that instead of strings you have numbers.
The easiest way to do such a thing is to create a mapping where:
red --> 1
yellow --> 2
blue --> 3
and replace each string with its mapped value.
However this might create unwanted side effects in our ML model as when dealing with numbers it might think that blue > yellow (because 3 > 2) or that red + yellow = blue (because 1 + 2 = 3). The model has no way of knowing that these data were categorical and then were mapped as integers.
The solution to this problem is one-hot encoding where we create N new features, where N is the number of unique values in the original feature. In our exampel N would be equal to 3, because we have 3 unique colors (red, yellow and blue).
Each of these features be binary and would correspond to one of these unique values. In our example the first feature would be a binary feature telling us if that sample is red or not, the second would be the same thing for yellow and the third for blue.
An example of such a transformation is illustrated below:
Note, that because this approach increases the dimensionality of the dataset, if we have a feature that takes many unique values, we may want to use a more sparse encoding (like the one I presented above).
$endgroup$
add a comment |
$begingroup$
Suppose you have a categorical feature in your dataset (e.g. color). And your samples can be either red, yellow or blue. In order to pass this argument to a ML algorithm, you first need to encode it so that instead of strings you have numbers.
The easiest way to do such a thing is to create a mapping where:
red --> 1
yellow --> 2
blue --> 3
and replace each string with its mapped value.
However this might create unwanted side effects in our ML model as when dealing with numbers it might think that blue > yellow (because 3 > 2) or that red + yellow = blue (because 1 + 2 = 3). The model has no way of knowing that these data were categorical and then were mapped as integers.
The solution to this problem is one-hot encoding where we create N new features, where N is the number of unique values in the original feature. In our exampel N would be equal to 3, because we have 3 unique colors (red, yellow and blue).
Each of these features be binary and would correspond to one of these unique values. In our example the first feature would be a binary feature telling us if that sample is red or not, the second would be the same thing for yellow and the third for blue.
An example of such a transformation is illustrated below:
Note, that because this approach increases the dimensionality of the dataset, if we have a feature that takes many unique values, we may want to use a more sparse encoding (like the one I presented above).
$endgroup$
Suppose you have a categorical feature in your dataset (e.g. color). And your samples can be either red, yellow or blue. In order to pass this argument to a ML algorithm, you first need to encode it so that instead of strings you have numbers.
The easiest way to do such a thing is to create a mapping where:
red --> 1
yellow --> 2
blue --> 3
and replace each string with its mapped value.
However this might create unwanted side effects in our ML model as when dealing with numbers it might think that blue > yellow (because 3 > 2) or that red + yellow = blue (because 1 + 2 = 3). The model has no way of knowing that these data were categorical and then were mapped as integers.
The solution to this problem is one-hot encoding where we create N new features, where N is the number of unique values in the original feature. In our exampel N would be equal to 3, because we have 3 unique colors (red, yellow and blue).
Each of these features be binary and would correspond to one of these unique values. In our example the first feature would be a binary feature telling us if that sample is red or not, the second would be the same thing for yellow and the third for blue.
An example of such a transformation is illustrated below:
Note, that because this approach increases the dimensionality of the dataset, if we have a feature that takes many unique values, we may want to use a more sparse encoding (like the one I presented above).
answered Apr 12 '18 at 10:05
Djib2011Djib2011
2,58231125
2,58231125
add a comment |
add a comment |
$begingroup$
depth: A scalar defining the depth of the one hot dimension.
indices: A Tensor of indices.
This the example given in tensorflow documentation.
1. Only Specifying indices and depth(Default Values of on_value is 1 and off_value is 0)
indices = [0, 1, 2]
depth = 3
tf.one_hot(indices, depth) # output: [3 x 3]
# [[1., 0., 0.],
# [0., 1., 0.],
# [0., 0., 1.]]
- Specifying on_value and off_value
indices = [0, 2, -1, 1]
depth = 3
tf.one_hot(indices, depth, on_value=5.0, off_value=0.0, axis=-1)
##output: [4 x 3]
# [[5.0, 0.0, 0.0], # one_hot(0)
# [0.0, 0.0, 5.0], # one_hot(2)
# [0.0, 0.0, 0.0], # one_hot(-1)
# [0.0, 5.0, 0.0]] # one_hot(1)
You can also see the code on GitHub
$endgroup$
add a comment |
$begingroup$
depth: A scalar defining the depth of the one hot dimension.
indices: A Tensor of indices.
This the example given in tensorflow documentation.
1. Only Specifying indices and depth(Default Values of on_value is 1 and off_value is 0)
indices = [0, 1, 2]
depth = 3
tf.one_hot(indices, depth) # output: [3 x 3]
# [[1., 0., 0.],
# [0., 1., 0.],
# [0., 0., 1.]]
- Specifying on_value and off_value
indices = [0, 2, -1, 1]
depth = 3
tf.one_hot(indices, depth, on_value=5.0, off_value=0.0, axis=-1)
##output: [4 x 3]
# [[5.0, 0.0, 0.0], # one_hot(0)
# [0.0, 0.0, 5.0], # one_hot(2)
# [0.0, 0.0, 0.0], # one_hot(-1)
# [0.0, 5.0, 0.0]] # one_hot(1)
You can also see the code on GitHub
$endgroup$
add a comment |
$begingroup$
depth: A scalar defining the depth of the one hot dimension.
indices: A Tensor of indices.
This the example given in tensorflow documentation.
1. Only Specifying indices and depth(Default Values of on_value is 1 and off_value is 0)
indices = [0, 1, 2]
depth = 3
tf.one_hot(indices, depth) # output: [3 x 3]
# [[1., 0., 0.],
# [0., 1., 0.],
# [0., 0., 1.]]
- Specifying on_value and off_value
indices = [0, 2, -1, 1]
depth = 3
tf.one_hot(indices, depth, on_value=5.0, off_value=0.0, axis=-1)
##output: [4 x 3]
# [[5.0, 0.0, 0.0], # one_hot(0)
# [0.0, 0.0, 5.0], # one_hot(2)
# [0.0, 0.0, 0.0], # one_hot(-1)
# [0.0, 5.0, 0.0]] # one_hot(1)
You can also see the code on GitHub
$endgroup$
depth: A scalar defining the depth of the one hot dimension.
indices: A Tensor of indices.
This the example given in tensorflow documentation.
1. Only Specifying indices and depth(Default Values of on_value is 1 and off_value is 0)
indices = [0, 1, 2]
depth = 3
tf.one_hot(indices, depth) # output: [3 x 3]
# [[1., 0., 0.],
# [0., 1., 0.],
# [0., 0., 1.]]
- Specifying on_value and off_value
indices = [0, 2, -1, 1]
depth = 3
tf.one_hot(indices, depth, on_value=5.0, off_value=0.0, axis=-1)
##output: [4 x 3]
# [[5.0, 0.0, 0.0], # one_hot(0)
# [0.0, 0.0, 5.0], # one_hot(2)
# [0.0, 0.0, 0.0], # one_hot(-1)
# [0.0, 5.0, 0.0]] # one_hot(1)
You can also see the code on GitHub
answered Apr 12 '18 at 12:05


VallieVallie
314
314
add a comment |
add a comment |
Thanks for contributing an answer to Data Science Stack Exchange!
- Please be sure to answer the question. Provide details and share your research!
But avoid …
- Asking for help, clarification, or responding to other answers.
- Making statements based on opinion; back them up with references or personal experience.
Use MathJax to format equations. MathJax reference.
To learn more, see our tips on writing great answers.
Sign up or log in
StackExchange.ready(function ()
StackExchange.helpers.onClickDraftSave('#login-link');
);
Sign up using Google
Sign up using Facebook
Sign up using Email and Password
Post as a guest
Required, but never shown
StackExchange.ready(
function ()
StackExchange.openid.initPostLogin('.new-post-login', 'https%3a%2f%2fdatascience.stackexchange.com%2fquestions%2f30215%2fwhat-is-one-hot-encoding-in-tensorflow%23new-answer', 'question_page');
);
Post as a guest
Required, but never shown
Sign up or log in
StackExchange.ready(function ()
StackExchange.helpers.onClickDraftSave('#login-link');
);
Sign up using Google
Sign up using Facebook
Sign up using Email and Password
Post as a guest
Required, but never shown
Sign up or log in
StackExchange.ready(function ()
StackExchange.helpers.onClickDraftSave('#login-link');
);
Sign up using Google
Sign up using Facebook
Sign up using Email and Password
Post as a guest
Required, but never shown
Sign up or log in
StackExchange.ready(function ()
StackExchange.helpers.onClickDraftSave('#login-link');
);
Sign up using Google
Sign up using Facebook
Sign up using Email and Password
Sign up using Google
Sign up using Facebook
Sign up using Email and Password
Post as a guest
Required, but never shown
Required, but never shown
Required, but never shown
Required, but never shown
Required, but never shown
Required, but never shown
Required, but never shown
Required, but never shown
Required, but never shown
n5,s,aJYXSkojZAQITzt,SW8kT