Genetic neural network to satisfy variable number of inputs and outputs Announcing the arrival of Valued Associate #679: Cesar Manara Planned maintenance scheduled April 23, 2019 at 23:30 UTC (7:30pm US/Eastern) 2019 Moderator Election Q&A - Questionnaire 2019 Community Moderator Election ResultsNeural network obfuscationCheckers playing Neural Network evolved with Genetic Algorithm becomes too sensitive to input data changesAn Artificial Neuron Network (ANN) with an arbitrary number of inputs and outputsHow to prevent a neural network from choosing the 'easiest' solutionUnevenly stretched sequences with LSTM/GRUEncog neural network multiple outputsText classification with neural network number of input neuronsContinuously predicting eventsMinimum Neurons in Neural NetworkUnderstanding a Neural Network with Keras (preferably), TensorFlow or PyTorch
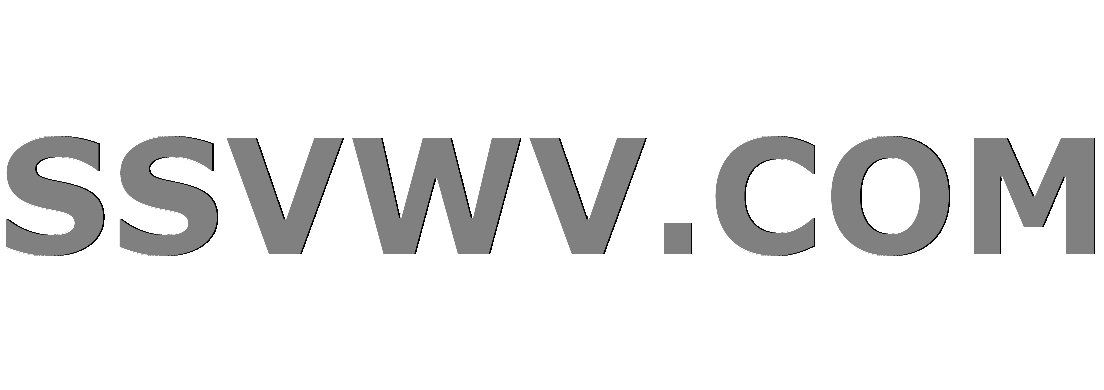
Multi tool use
Why does BitLocker not use RSA?
Problem with display of presentation
What should one know about term logic before studying propositional and predicate logic?
Dinosaur Word Search, Letter Solve, and Unscramble
How does the body cool itself in a stillsuit?
The test team as an enemy of development? And how can this be avoided?
Noise in Eigenvalues plot
Determine whether an integer is a palindrome
Meaning of 境 in その日を境に
How many time has Arya actually used Needle?
How to make an animal which can only breed for a certain number of generations?
Why not use the yoke to control yaw, as well as pitch and roll?
Pointing to problems without suggesting solutions
French equivalents of おしゃれは足元から (Every good outfit starts with the shoes)
Inverse square law not accurate for non-point masses?
Is the time—manner—place ordering of adverbials an oversimplification?
How could a hydrazine and N2O4 cloud (or it's reactants) show up in weather radar?
Is a copyright notice with a non-existent name be invalid?
How do you cope with tons of web fonts when copying and pasting from web pages?
How to make triangles with rounded sides and corners? (squircle with 3 sides)
How do Java 8 default methods hеlp with lambdas?
Short story about astronauts fertilizing soil with their own bodies
Understanding piped commands in GNU/Linux
How do I say "this must not happen"?
Genetic neural network to satisfy variable number of inputs and outputs
Announcing the arrival of Valued Associate #679: Cesar Manara
Planned maintenance scheduled April 23, 2019 at 23:30 UTC (7:30pm US/Eastern)
2019 Moderator Election Q&A - Questionnaire
2019 Community Moderator Election ResultsNeural network obfuscationCheckers playing Neural Network evolved with Genetic Algorithm becomes too sensitive to input data changesAn Artificial Neuron Network (ANN) with an arbitrary number of inputs and outputsHow to prevent a neural network from choosing the 'easiest' solutionUnevenly stretched sequences with LSTM/GRUEncog neural network multiple outputsText classification with neural network number of input neuronsContinuously predicting eventsMinimum Neurons in Neural NetworkUnderstanding a Neural Network with Keras (preferably), TensorFlow or PyTorch
$begingroup$
I have what I propose as a solution to my problem, however I haven't ever seen it mentioned in this way, so I worry that there is a valid reason not to do things this way.
I have a dataset of > 100,000 events, where each event has a winner.
I have plenty of data points, some data on the event itself, and some data on each entrant.
The number of entrants in each event is variable, and I want to build a neural network around picking a likely winner of the events.
As the number of entrants is variable, what appears to be common advice is to have enough inputs for the maximum case scenario, and 0 them out for events where there are empty slots.
This feels somewhat inelegant, and I had a slightly different idea.
I was going to have a NN where the inputs are information about the event, and information about 1 entrant. I would then have a single output (a float between 0 and 1). I would run this through, getting 1 output for each entrant in an event, then I would be left with a number of floats, equal to the number of entrants in the event. I would then select the highest value, and use the entrant that refers to as the choice for the winner.
Is there a reason I shouldn't be doing it this way? Is there a better solution I haven't yet come across?
neural-network dataset genetic-algorithms
$endgroup$
bumped to the homepage by Community♦ 33 mins ago
This question has answers that may be good or bad; the system has marked it active so that they can be reviewed.
add a comment |
$begingroup$
I have what I propose as a solution to my problem, however I haven't ever seen it mentioned in this way, so I worry that there is a valid reason not to do things this way.
I have a dataset of > 100,000 events, where each event has a winner.
I have plenty of data points, some data on the event itself, and some data on each entrant.
The number of entrants in each event is variable, and I want to build a neural network around picking a likely winner of the events.
As the number of entrants is variable, what appears to be common advice is to have enough inputs for the maximum case scenario, and 0 them out for events where there are empty slots.
This feels somewhat inelegant, and I had a slightly different idea.
I was going to have a NN where the inputs are information about the event, and information about 1 entrant. I would then have a single output (a float between 0 and 1). I would run this through, getting 1 output for each entrant in an event, then I would be left with a number of floats, equal to the number of entrants in the event. I would then select the highest value, and use the entrant that refers to as the choice for the winner.
Is there a reason I shouldn't be doing it this way? Is there a better solution I haven't yet come across?
neural-network dataset genetic-algorithms
$endgroup$
bumped to the homepage by Community♦ 33 mins ago
This question has answers that may be good or bad; the system has marked it active so that they can be reviewed.
1
$begingroup$
Why are you using "Genetic" in the title, and thegenetic-algorithms
tag? I cannot see the link . . . is the intent that you want this to be a genetic algorithm, or can you explain why you think it is one?
$endgroup$
– Neil Slater
Sep 26 '17 at 14:33
$begingroup$
I plan to have these NN's randomly assign weights from each neuron to start, then assess their fitness across my training dataset and kill off half, and crossover the other half to create generation 2, and repeat until I either see no progress for an extended period of time or hit a desired result.
$endgroup$
– pingu2k4
Sep 26 '17 at 14:37
1
$begingroup$
OK, right. I don't think that is relevant to the question, as it is not about training your model. Probably worth adding that detail in the question, maybe alter the title to make it focus on your problem - whether to have one multiple input/output network or run a simpler network multiple times . . . I don't think it matters hugely how it will be trained (although beware genetic algorithms don't scale well in NNs - if your NN becomes large/complex, a GA may struggle to find optimums)
$endgroup$
– Neil Slater
Sep 26 '17 at 14:40
add a comment |
$begingroup$
I have what I propose as a solution to my problem, however I haven't ever seen it mentioned in this way, so I worry that there is a valid reason not to do things this way.
I have a dataset of > 100,000 events, where each event has a winner.
I have plenty of data points, some data on the event itself, and some data on each entrant.
The number of entrants in each event is variable, and I want to build a neural network around picking a likely winner of the events.
As the number of entrants is variable, what appears to be common advice is to have enough inputs for the maximum case scenario, and 0 them out for events where there are empty slots.
This feels somewhat inelegant, and I had a slightly different idea.
I was going to have a NN where the inputs are information about the event, and information about 1 entrant. I would then have a single output (a float between 0 and 1). I would run this through, getting 1 output for each entrant in an event, then I would be left with a number of floats, equal to the number of entrants in the event. I would then select the highest value, and use the entrant that refers to as the choice for the winner.
Is there a reason I shouldn't be doing it this way? Is there a better solution I haven't yet come across?
neural-network dataset genetic-algorithms
$endgroup$
I have what I propose as a solution to my problem, however I haven't ever seen it mentioned in this way, so I worry that there is a valid reason not to do things this way.
I have a dataset of > 100,000 events, where each event has a winner.
I have plenty of data points, some data on the event itself, and some data on each entrant.
The number of entrants in each event is variable, and I want to build a neural network around picking a likely winner of the events.
As the number of entrants is variable, what appears to be common advice is to have enough inputs for the maximum case scenario, and 0 them out for events where there are empty slots.
This feels somewhat inelegant, and I had a slightly different idea.
I was going to have a NN where the inputs are information about the event, and information about 1 entrant. I would then have a single output (a float between 0 and 1). I would run this through, getting 1 output for each entrant in an event, then I would be left with a number of floats, equal to the number of entrants in the event. I would then select the highest value, and use the entrant that refers to as the choice for the winner.
Is there a reason I shouldn't be doing it this way? Is there a better solution I haven't yet come across?
neural-network dataset genetic-algorithms
neural-network dataset genetic-algorithms
asked Sep 26 '17 at 14:27
pingu2k4pingu2k4
1141
1141
bumped to the homepage by Community♦ 33 mins ago
This question has answers that may be good or bad; the system has marked it active so that they can be reviewed.
bumped to the homepage by Community♦ 33 mins ago
This question has answers that may be good or bad; the system has marked it active so that they can be reviewed.
1
$begingroup$
Why are you using "Genetic" in the title, and thegenetic-algorithms
tag? I cannot see the link . . . is the intent that you want this to be a genetic algorithm, or can you explain why you think it is one?
$endgroup$
– Neil Slater
Sep 26 '17 at 14:33
$begingroup$
I plan to have these NN's randomly assign weights from each neuron to start, then assess their fitness across my training dataset and kill off half, and crossover the other half to create generation 2, and repeat until I either see no progress for an extended period of time or hit a desired result.
$endgroup$
– pingu2k4
Sep 26 '17 at 14:37
1
$begingroup$
OK, right. I don't think that is relevant to the question, as it is not about training your model. Probably worth adding that detail in the question, maybe alter the title to make it focus on your problem - whether to have one multiple input/output network or run a simpler network multiple times . . . I don't think it matters hugely how it will be trained (although beware genetic algorithms don't scale well in NNs - if your NN becomes large/complex, a GA may struggle to find optimums)
$endgroup$
– Neil Slater
Sep 26 '17 at 14:40
add a comment |
1
$begingroup$
Why are you using "Genetic" in the title, and thegenetic-algorithms
tag? I cannot see the link . . . is the intent that you want this to be a genetic algorithm, or can you explain why you think it is one?
$endgroup$
– Neil Slater
Sep 26 '17 at 14:33
$begingroup$
I plan to have these NN's randomly assign weights from each neuron to start, then assess their fitness across my training dataset and kill off half, and crossover the other half to create generation 2, and repeat until I either see no progress for an extended period of time or hit a desired result.
$endgroup$
– pingu2k4
Sep 26 '17 at 14:37
1
$begingroup$
OK, right. I don't think that is relevant to the question, as it is not about training your model. Probably worth adding that detail in the question, maybe alter the title to make it focus on your problem - whether to have one multiple input/output network or run a simpler network multiple times . . . I don't think it matters hugely how it will be trained (although beware genetic algorithms don't scale well in NNs - if your NN becomes large/complex, a GA may struggle to find optimums)
$endgroup$
– Neil Slater
Sep 26 '17 at 14:40
1
1
$begingroup$
Why are you using "Genetic" in the title, and the
genetic-algorithms
tag? I cannot see the link . . . is the intent that you want this to be a genetic algorithm, or can you explain why you think it is one?$endgroup$
– Neil Slater
Sep 26 '17 at 14:33
$begingroup$
Why are you using "Genetic" in the title, and the
genetic-algorithms
tag? I cannot see the link . . . is the intent that you want this to be a genetic algorithm, or can you explain why you think it is one?$endgroup$
– Neil Slater
Sep 26 '17 at 14:33
$begingroup$
I plan to have these NN's randomly assign weights from each neuron to start, then assess their fitness across my training dataset and kill off half, and crossover the other half to create generation 2, and repeat until I either see no progress for an extended period of time or hit a desired result.
$endgroup$
– pingu2k4
Sep 26 '17 at 14:37
$begingroup$
I plan to have these NN's randomly assign weights from each neuron to start, then assess their fitness across my training dataset and kill off half, and crossover the other half to create generation 2, and repeat until I either see no progress for an extended period of time or hit a desired result.
$endgroup$
– pingu2k4
Sep 26 '17 at 14:37
1
1
$begingroup$
OK, right. I don't think that is relevant to the question, as it is not about training your model. Probably worth adding that detail in the question, maybe alter the title to make it focus on your problem - whether to have one multiple input/output network or run a simpler network multiple times . . . I don't think it matters hugely how it will be trained (although beware genetic algorithms don't scale well in NNs - if your NN becomes large/complex, a GA may struggle to find optimums)
$endgroup$
– Neil Slater
Sep 26 '17 at 14:40
$begingroup$
OK, right. I don't think that is relevant to the question, as it is not about training your model. Probably worth adding that detail in the question, maybe alter the title to make it focus on your problem - whether to have one multiple input/output network or run a simpler network multiple times . . . I don't think it matters hugely how it will be trained (although beware genetic algorithms don't scale well in NNs - if your NN becomes large/complex, a GA may struggle to find optimums)
$endgroup$
– Neil Slater
Sep 26 '17 at 14:40
add a comment |
2 Answers
2
active
oldest
votes
$begingroup$
Is there a reason I shouldn't be doing it this way?
Depends on the nature of the data. There might be an element of "Scissor/Paper/Stone" in the competition you are scoring, where different strengths and weaknesses of competitors can combine such that Player A beats Player B, Player B beats Player C, but Player C beats Player A. In that case, you cannot produce reliable ranking between players by considering each entrant separately, and a network that rates each player individually will perform less well than one that can compare players.
If players are in more of a race-to-finish or score max points separately in a competition, then separately rating each player in each competition should be more reliable. And it is definitely easier to build and train a neural network to predict that.
An alternative, if your events are more like tournaments where entrants oppose each other (even if within some larger free-for-all), is to predict relative rank between pairs of players. This may not be consistent, so you will need to use a pairwise ranking method to resolve that for the final winner. If it really is a knockout tournament, and you know how the initial draw and team combinations will work, then you could maybe make a prediction by simulating the possible games.
There is nothing preventing you from combining these approaches in some way either.
Whichever method you use, you will want to think a little about what your metric is going to be to select the best approach. If you only care about predicting the winner, then accuracy of that prediction might be enough. If you care about where the eventual winner is placed, perhaps mean reciprocal rank would be better (score 1 for correct prediction, 1/2 for predicting winner as ranked second, 1/3 if third etc).
$endgroup$
add a comment |
$begingroup$
I have taken a deep foray into the world of genetic algorithms and think that your inclusion of this tag may not be readily apparent in your question, but inadvertently may provide the best solution to your problem.
I would suggest using a implementation of either hyperneat, or es-hyperneat, both of which evolve genotype cppns that in turn build phenotype neural network substrates, if you train and evolve your cppn with variable numbers of inputs I would suspect the cppn to evolve and to account for that (this may be by grouping inputs to create subnets, who knows). I currently use this to solve a similar problem that also has variable number of inputs, as long as you don't have a variable number of dimensions in your node layouts (im not sure how this could even happen) you should be able to use these algorithms.
$endgroup$
add a comment |
Your Answer
StackExchange.ready(function()
var channelOptions =
tags: "".split(" "),
id: "557"
;
initTagRenderer("".split(" "), "".split(" "), channelOptions);
StackExchange.using("externalEditor", function()
// Have to fire editor after snippets, if snippets enabled
if (StackExchange.settings.snippets.snippetsEnabled)
StackExchange.using("snippets", function()
createEditor();
);
else
createEditor();
);
function createEditor()
StackExchange.prepareEditor(
heartbeatType: 'answer',
autoActivateHeartbeat: false,
convertImagesToLinks: false,
noModals: true,
showLowRepImageUploadWarning: true,
reputationToPostImages: null,
bindNavPrevention: true,
postfix: "",
imageUploader:
brandingHtml: "Powered by u003ca class="icon-imgur-white" href="https://imgur.com/"u003eu003c/au003e",
contentPolicyHtml: "User contributions licensed under u003ca href="https://creativecommons.org/licenses/by-sa/3.0/"u003ecc by-sa 3.0 with attribution requiredu003c/au003e u003ca href="https://stackoverflow.com/legal/content-policy"u003e(content policy)u003c/au003e",
allowUrls: true
,
onDemand: true,
discardSelector: ".discard-answer"
,immediatelyShowMarkdownHelp:true
);
);
Sign up or log in
StackExchange.ready(function ()
StackExchange.helpers.onClickDraftSave('#login-link');
);
Sign up using Google
Sign up using Facebook
Sign up using Email and Password
Post as a guest
Required, but never shown
StackExchange.ready(
function ()
StackExchange.openid.initPostLogin('.new-post-login', 'https%3a%2f%2fdatascience.stackexchange.com%2fquestions%2f23334%2fgenetic-neural-network-to-satisfy-variable-number-of-inputs-and-outputs%23new-answer', 'question_page');
);
Post as a guest
Required, but never shown
2 Answers
2
active
oldest
votes
2 Answers
2
active
oldest
votes
active
oldest
votes
active
oldest
votes
$begingroup$
Is there a reason I shouldn't be doing it this way?
Depends on the nature of the data. There might be an element of "Scissor/Paper/Stone" in the competition you are scoring, where different strengths and weaknesses of competitors can combine such that Player A beats Player B, Player B beats Player C, but Player C beats Player A. In that case, you cannot produce reliable ranking between players by considering each entrant separately, and a network that rates each player individually will perform less well than one that can compare players.
If players are in more of a race-to-finish or score max points separately in a competition, then separately rating each player in each competition should be more reliable. And it is definitely easier to build and train a neural network to predict that.
An alternative, if your events are more like tournaments where entrants oppose each other (even if within some larger free-for-all), is to predict relative rank between pairs of players. This may not be consistent, so you will need to use a pairwise ranking method to resolve that for the final winner. If it really is a knockout tournament, and you know how the initial draw and team combinations will work, then you could maybe make a prediction by simulating the possible games.
There is nothing preventing you from combining these approaches in some way either.
Whichever method you use, you will want to think a little about what your metric is going to be to select the best approach. If you only care about predicting the winner, then accuracy of that prediction might be enough. If you care about where the eventual winner is placed, perhaps mean reciprocal rank would be better (score 1 for correct prediction, 1/2 for predicting winner as ranked second, 1/3 if third etc).
$endgroup$
add a comment |
$begingroup$
Is there a reason I shouldn't be doing it this way?
Depends on the nature of the data. There might be an element of "Scissor/Paper/Stone" in the competition you are scoring, where different strengths and weaknesses of competitors can combine such that Player A beats Player B, Player B beats Player C, but Player C beats Player A. In that case, you cannot produce reliable ranking between players by considering each entrant separately, and a network that rates each player individually will perform less well than one that can compare players.
If players are in more of a race-to-finish or score max points separately in a competition, then separately rating each player in each competition should be more reliable. And it is definitely easier to build and train a neural network to predict that.
An alternative, if your events are more like tournaments where entrants oppose each other (even if within some larger free-for-all), is to predict relative rank between pairs of players. This may not be consistent, so you will need to use a pairwise ranking method to resolve that for the final winner. If it really is a knockout tournament, and you know how the initial draw and team combinations will work, then you could maybe make a prediction by simulating the possible games.
There is nothing preventing you from combining these approaches in some way either.
Whichever method you use, you will want to think a little about what your metric is going to be to select the best approach. If you only care about predicting the winner, then accuracy of that prediction might be enough. If you care about where the eventual winner is placed, perhaps mean reciprocal rank would be better (score 1 for correct prediction, 1/2 for predicting winner as ranked second, 1/3 if third etc).
$endgroup$
add a comment |
$begingroup$
Is there a reason I shouldn't be doing it this way?
Depends on the nature of the data. There might be an element of "Scissor/Paper/Stone" in the competition you are scoring, where different strengths and weaknesses of competitors can combine such that Player A beats Player B, Player B beats Player C, but Player C beats Player A. In that case, you cannot produce reliable ranking between players by considering each entrant separately, and a network that rates each player individually will perform less well than one that can compare players.
If players are in more of a race-to-finish or score max points separately in a competition, then separately rating each player in each competition should be more reliable. And it is definitely easier to build and train a neural network to predict that.
An alternative, if your events are more like tournaments where entrants oppose each other (even if within some larger free-for-all), is to predict relative rank between pairs of players. This may not be consistent, so you will need to use a pairwise ranking method to resolve that for the final winner. If it really is a knockout tournament, and you know how the initial draw and team combinations will work, then you could maybe make a prediction by simulating the possible games.
There is nothing preventing you from combining these approaches in some way either.
Whichever method you use, you will want to think a little about what your metric is going to be to select the best approach. If you only care about predicting the winner, then accuracy of that prediction might be enough. If you care about where the eventual winner is placed, perhaps mean reciprocal rank would be better (score 1 for correct prediction, 1/2 for predicting winner as ranked second, 1/3 if third etc).
$endgroup$
Is there a reason I shouldn't be doing it this way?
Depends on the nature of the data. There might be an element of "Scissor/Paper/Stone" in the competition you are scoring, where different strengths and weaknesses of competitors can combine such that Player A beats Player B, Player B beats Player C, but Player C beats Player A. In that case, you cannot produce reliable ranking between players by considering each entrant separately, and a network that rates each player individually will perform less well than one that can compare players.
If players are in more of a race-to-finish or score max points separately in a competition, then separately rating each player in each competition should be more reliable. And it is definitely easier to build and train a neural network to predict that.
An alternative, if your events are more like tournaments where entrants oppose each other (even if within some larger free-for-all), is to predict relative rank between pairs of players. This may not be consistent, so you will need to use a pairwise ranking method to resolve that for the final winner. If it really is a knockout tournament, and you know how the initial draw and team combinations will work, then you could maybe make a prediction by simulating the possible games.
There is nothing preventing you from combining these approaches in some way either.
Whichever method you use, you will want to think a little about what your metric is going to be to select the best approach. If you only care about predicting the winner, then accuracy of that prediction might be enough. If you care about where the eventual winner is placed, perhaps mean reciprocal rank would be better (score 1 for correct prediction, 1/2 for predicting winner as ranked second, 1/3 if third etc).
edited Sep 26 '17 at 16:03
answered Sep 26 '17 at 15:07
Neil SlaterNeil Slater
17.8k33264
17.8k33264
add a comment |
add a comment |
$begingroup$
I have taken a deep foray into the world of genetic algorithms and think that your inclusion of this tag may not be readily apparent in your question, but inadvertently may provide the best solution to your problem.
I would suggest using a implementation of either hyperneat, or es-hyperneat, both of which evolve genotype cppns that in turn build phenotype neural network substrates, if you train and evolve your cppn with variable numbers of inputs I would suspect the cppn to evolve and to account for that (this may be by grouping inputs to create subnets, who knows). I currently use this to solve a similar problem that also has variable number of inputs, as long as you don't have a variable number of dimensions in your node layouts (im not sure how this could even happen) you should be able to use these algorithms.
$endgroup$
add a comment |
$begingroup$
I have taken a deep foray into the world of genetic algorithms and think that your inclusion of this tag may not be readily apparent in your question, but inadvertently may provide the best solution to your problem.
I would suggest using a implementation of either hyperneat, or es-hyperneat, both of which evolve genotype cppns that in turn build phenotype neural network substrates, if you train and evolve your cppn with variable numbers of inputs I would suspect the cppn to evolve and to account for that (this may be by grouping inputs to create subnets, who knows). I currently use this to solve a similar problem that also has variable number of inputs, as long as you don't have a variable number of dimensions in your node layouts (im not sure how this could even happen) you should be able to use these algorithms.
$endgroup$
add a comment |
$begingroup$
I have taken a deep foray into the world of genetic algorithms and think that your inclusion of this tag may not be readily apparent in your question, but inadvertently may provide the best solution to your problem.
I would suggest using a implementation of either hyperneat, or es-hyperneat, both of which evolve genotype cppns that in turn build phenotype neural network substrates, if you train and evolve your cppn with variable numbers of inputs I would suspect the cppn to evolve and to account for that (this may be by grouping inputs to create subnets, who knows). I currently use this to solve a similar problem that also has variable number of inputs, as long as you don't have a variable number of dimensions in your node layouts (im not sure how this could even happen) you should be able to use these algorithms.
$endgroup$
I have taken a deep foray into the world of genetic algorithms and think that your inclusion of this tag may not be readily apparent in your question, but inadvertently may provide the best solution to your problem.
I would suggest using a implementation of either hyperneat, or es-hyperneat, both of which evolve genotype cppns that in turn build phenotype neural network substrates, if you train and evolve your cppn with variable numbers of inputs I would suspect the cppn to evolve and to account for that (this may be by grouping inputs to create subnets, who knows). I currently use this to solve a similar problem that also has variable number of inputs, as long as you don't have a variable number of dimensions in your node layouts (im not sure how this could even happen) you should be able to use these algorithms.
edited Nov 19 '18 at 22:37


Stephen Rauch♦
1,52551330
1,52551330
answered Nov 19 '18 at 17:58


nickwnickw
11
11
add a comment |
add a comment |
Thanks for contributing an answer to Data Science Stack Exchange!
- Please be sure to answer the question. Provide details and share your research!
But avoid …
- Asking for help, clarification, or responding to other answers.
- Making statements based on opinion; back them up with references or personal experience.
Use MathJax to format equations. MathJax reference.
To learn more, see our tips on writing great answers.
Sign up or log in
StackExchange.ready(function ()
StackExchange.helpers.onClickDraftSave('#login-link');
);
Sign up using Google
Sign up using Facebook
Sign up using Email and Password
Post as a guest
Required, but never shown
StackExchange.ready(
function ()
StackExchange.openid.initPostLogin('.new-post-login', 'https%3a%2f%2fdatascience.stackexchange.com%2fquestions%2f23334%2fgenetic-neural-network-to-satisfy-variable-number-of-inputs-and-outputs%23new-answer', 'question_page');
);
Post as a guest
Required, but never shown
Sign up or log in
StackExchange.ready(function ()
StackExchange.helpers.onClickDraftSave('#login-link');
);
Sign up using Google
Sign up using Facebook
Sign up using Email and Password
Post as a guest
Required, but never shown
Sign up or log in
StackExchange.ready(function ()
StackExchange.helpers.onClickDraftSave('#login-link');
);
Sign up using Google
Sign up using Facebook
Sign up using Email and Password
Post as a guest
Required, but never shown
Sign up or log in
StackExchange.ready(function ()
StackExchange.helpers.onClickDraftSave('#login-link');
);
Sign up using Google
Sign up using Facebook
Sign up using Email and Password
Sign up using Google
Sign up using Facebook
Sign up using Email and Password
Post as a guest
Required, but never shown
Required, but never shown
Required, but never shown
Required, but never shown
Required, but never shown
Required, but never shown
Required, but never shown
Required, but never shown
Required, but never shown
2,uG o OtVre p4qxYd4B,emV5T QDK iMEaV 7M8gdm,ZUdB p8kR7BNXbNlcobVT
1
$begingroup$
Why are you using "Genetic" in the title, and the
genetic-algorithms
tag? I cannot see the link . . . is the intent that you want this to be a genetic algorithm, or can you explain why you think it is one?$endgroup$
– Neil Slater
Sep 26 '17 at 14:33
$begingroup$
I plan to have these NN's randomly assign weights from each neuron to start, then assess their fitness across my training dataset and kill off half, and crossover the other half to create generation 2, and repeat until I either see no progress for an extended period of time or hit a desired result.
$endgroup$
– pingu2k4
Sep 26 '17 at 14:37
1
$begingroup$
OK, right. I don't think that is relevant to the question, as it is not about training your model. Probably worth adding that detail in the question, maybe alter the title to make it focus on your problem - whether to have one multiple input/output network or run a simpler network multiple times . . . I don't think it matters hugely how it will be trained (although beware genetic algorithms don't scale well in NNs - if your NN becomes large/complex, a GA may struggle to find optimums)
$endgroup$
– Neil Slater
Sep 26 '17 at 14:40